Scientists have developed mathematical models that analyze the data obtained from an initial wave of the influenza pandemic to predict the dynamics of subsequent waves. Such predictions could be useful for pandemic preparedness.
The study has been published in PLOS COMPUTATIONAL BIOLOGY.
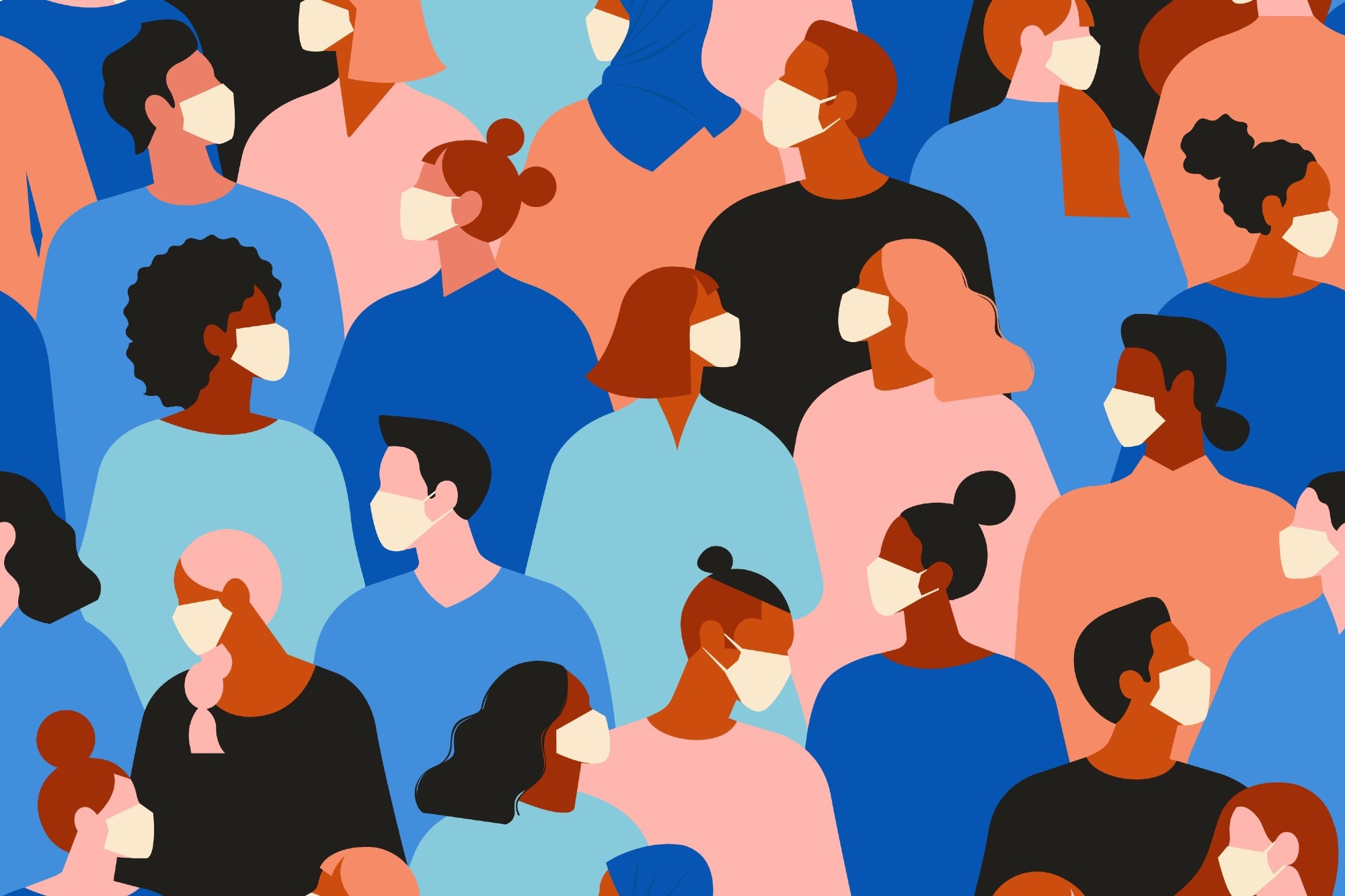
Background
The influenza pandemic caused by H1N1 influenza virus in 1918 took approximately 50 million lives worldwide. The case fatality rate of the influenza pandemic was similar to that estimated during the ongoing coronavirus disease 2019 (COVID-19) pandemic caused by severe acute respiratory syndrome coronavirus 2 (SARS-CoV-2).
Some highly pathogenic avian influenza viruses have continued to cause sporadic human infections in recent times. Although human-to-human transmission of these infections occurs occasionally, there remains a risk for the future emergence of a large-scale influenza pandemic.
Influenza infections generally follow a seasonal pattern, with already-identified viruses causing a wave of infections each winter in temperate countries across the world. Certain seasonal drivers, such as absolute humidity, play a vital role in shaping the dynamics of the influenza pandemic as well as seasonal influenza waves.
Influenza epidemics are mostly associated with an initial emergence of a novel viral variant, followed by a resurgence accompanying the onset of the annual influenza season. In the USA, the novel H1N1 influenza variant caused a “spring wave” between April and July 2009, with over 5 million people experiencing symptomatic infections.
Subsequently, a strong resurgence of the virus was observed during seasonal influenza infections in October 2009. This “fall wave” had caused an estimated 60.8 million infections and 274,304 hospitalizations by April 2010.
In the current study, scientists have analyzed the surveillance data obtained from the “spring wave” using mathematical models to predict the peak timing and health outcomes of the subsequent “fall wave.” They have applied this framework to guide the decision-making for future pandemics.
Important observations
The influenza infection surveillance data of the “spring wave” in 2009 was collected from ten states in the USA. The data was analyzed using mathematical models to predict the health outcomes of the “fall wave.” The effects of school opening as well as environmental triggers, were considered in the model.
In the surveillance data, many states, including California, showed clear signs of distinct spring and fall waves. The model used this data for the proportion of symptomatic cases that were laboratory-confirmed and hospitalized.
In contrast to California surveillance data, incomplete datasets of the spring wave were obtained from the states of Georgia, New Mexico, and Tennessee. These states also reported a significantly lower number of cases during the fall wave.
Using the surveillance data, the model predicted the peak timing and size of the fall wave. The model-predicted peak timing was slightly earlier than the actual fall wave peak timing documented. However, regarding the size of fall wave, the model predictions were reasonably comparable to the surveillance data.
Regarding the proportion of hospitalization during the fall wave, the model predictions were similar to the surveillance data for seven out of ten states studied. However, for the states of Georgia, New Mexico and Tennessee, the predictions were less accurate. Specifically, the model significantly overestimated the proportion of hospitalizations for these states. This could be due to insufficient surveillance data available for these states.
Application of the model
The scientists determined how this model can be applied for the timely prevention of upcoming waves. They specifically focused on preemptive school closures until a vaccine becomes available.
Considering a situation where 25% of the population is vaccinated, and preemptive school closures are implemented, the model predicted a significant reduction in health burden during the fall wave. According to the model prediction, preemptive school closures for ten weeks can lead to a 72% reduction in the proportion of hospitalization during the fall wave.
Study significance
The study describes how mathematical models of influenza transmission dynamics can be used to analyze pre-existing surveillance data obtained at the initial pandemic phase for future pandemic preparedness.
For the states with sufficient first wave surveillance data, the model accurately predicts the health burden of the second wave. However, the model fails to predict the peak timing of the second wave accurately.
- Haw DJ. (2023). Using real-time data to guide decision-making during an influenza pandemic: A modelling analysis. PLOS COMPUTATIONAL BIOLOGY. doi: https://doi.org/10.1371/journal.pcbi.1010893 https://journals.plos.org/ploscompbiol/article?id=10.1371/journal.pcbi.1010893
Posted in: Medical Research News | Miscellaneous News
Tags: Avian Influenza, Coronavirus, covid-19, H1N1, Influenza, Laboratory, Pandemic, Respiratory, SARS, SARS-CoV-2, Severe Acute Respiratory, Severe Acute Respiratory Syndrome, Syndrome, Vaccine, Virus
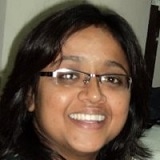
Written by
Dr. Sanchari Sinha Dutta
Dr. Sanchari Sinha Dutta is a science communicator who believes in spreading the power of science in every corner of the world. She has a Bachelor of Science (B.Sc.) degree and a Master's of Science (M.Sc.) in biology and human physiology. Following her Master's degree, Sanchari went on to study a Ph.D. in human physiology. She has authored more than 10 original research articles, all of which have been published in world renowned international journals.
Source: Read Full Article